skailark: Overcoming the Challenge of
Cost Competitive Intelligence
December 2021
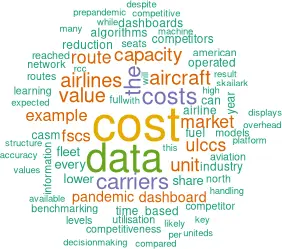
Airline leaders are experiencing fundamental changes in demand patterns in the wake of the pandemic. With new remote work options and an increase in environmental awareness, some of those changes are likely to stay. Network planning and revenue steering departments are in a trial-and-error mode like never before.
Additionally, the pandemic has left lasting impact on airline costs. Various survival strategies have left airlines with significantly different fleet and staff utilisation — and they are recovering at different rates. The pre-pandemic pilot shortage is already coming back on the agenda in parts of the world. A return to full capacity associated with expected post-pandemic growth is likely to drive salaries in this area. And, how will the requirement for thoroughly cleaned/disinfected aircraft and gate areas develop? Will it vanish post pandemic or continue to stay as part of the handling and airport bill?
Other cost-influencing trends have been accelerated, such as the early retirement of aircraft with high operating costs. With the industry’s goal for carbon neutrality by 2050, the trend of accelerated fleet renewal cycles is likely to continue. Connected to this, CORSIA and other carbon offsetting schemes will add to fuel-related costs. At the same time, cost pressures are returning quickly with Ultra Low Cost Carriers (ULCCs) recovering their capacities fastest.
Many airlines have received financial state support throughout the pandemic, including some that would have been expected to collapse. Therefore, a catch-up effect regarding consolidation (especially in Europe) is to be expected, possibly driving scale effects but also integration expenses.
Issue of data availability
As a result, understanding how an airline’s cost structure compares to its competitors has hardly ever been more critical. However, the competitive cost analytics capabilities across airline strategy, fleet, network, and investor relations departments remain somewhat confined today. Reasons for this include constrained competitive data availability and reporting depth as well as varying accounting approaches that limit comparability.
Even in the US market, where the Department of Transportation (DoT) publishes extensive (albeit aggregated) cost figures, airlines struggle clearly to identify how their cost structure compares to their competitors on a like-for-like basis. Furthermore, substantial top-down assumptions based on ‘industry expertise’ (like the split of cost between wide-bodied and narrow-bodied aircraft) drive major differences. Often, those assumptions culminate in self-fulfilling prophecies, far from the actual truth of the underlying costs.
While the whole aviation industry produces vast amounts of data every second of every day (think aircraft positioning data or real-time engine monitoring), such data is never fully aggregated into a useful format to allow for better cost benchmarking and decision-making. Imagining a world with transparent knowledge of the industry’s cost structure, would a proper cost data and benchmarking set have prevented many airlines from purchasing the A380 outright? Also, how would the network planning process change if accurate cost data of all competitors’ airline-aircraft combinations on all routes could be included in the decision-making process?
The solution: holistic airline cost data
A start-up called skailark has taken up the cause. The team is developing a bottom-up data image of the global aviation industry with the intention to provide better cost and market intelligence to airlines, their suppliers and service providers. What differentiates them from previous attempts is their holistic big data approach. Based on industry experience, academic research and machine learning algorithms, the team is creating a model of almost every aspect of the aviation value chain. This includes over 100 distinct but connected models to estimate, for example, the fuel consumption on every flight globally, or the shop visit schedule and cost for every engine in the market (incl spares).
Machine learning models are implemented to steadily improve the data accuracy through the ever-increasing data base over time. Already today, the data image created for North American airlines has reached over 97% accuracy compared with publicly reported information.
The machine learning models are used to further optimise accuracy over time. Historically estimated data acts as training data for the algorithms. The selection of the right algorithm depends on the actual use case and data properties. The data presents itself mostly as panel or longitudinal data since many of the values are observed across sections (eg airlines) and time. Boundary conditions are integrated and are generally based on industry experience (eg minimum aircraft handling cost per turn). Predominantly, supervised learning algorithms are applied to date, enabling the data scientists to nudge algorithms into the right direction. As a result, based on historic developments, the models predict values for situations that have not yet been observed, or can potentially forecast data into the future.
With such databases and algorithms there are plenty of possible applications. Skailark’s first product is a cost benchmarking and modelling platform. On this platform, all cost data are provided and visualised through web-based dashboards. These dashboards enable a straight-forward assessment of the data at any level of granularity. Furthermore, dynamic scenario-modelling capabilities facilitate decision-making. Chart 1 demonstrates a mock-up of an example dashboard, showing unit cost (CASM) development over time by cost type. While this view illustrates North American Full Service Carriers (FSCs), the platform includes the data for the 200 plus largest airlines globally. Various drop-down menus and selectors displayed on this dashboard allow adjustments of the cost data, enabling, for example, route- or aircraft-specific benchmarks. The additional data showcased below the bar charts is used for the interpretation of the results.
Example 1: Cost performance during the pandemic
As an example, this cost benchmarking dashboard output assesses the cost performance of North American carriers throughout the pandemic. Charts 2 and 3, respectively, display mock-ups of the above unit cost time-series dashboard for Value carriers such as JetBlue and Alaska, and for ULCCs such as Spirit and Frontier.
Based on the three outputs we can make some key observations of how the carrier types were handling capacity levels. Comparing the capacity reduction (in ASM) between Q2’20 and Q2’19, FSCs exhibited the highest reduction of 82% year on year (-78% for their narrow-bodied fleet), Value carriers of 55% and ULCCs of 38%. Of course, a key driver for the large capacity reduction of FSCs is the elimination of intercontinental traffic, which is mostly served by their wide-bodied aircraft. Nonetheless, the reduction of narrowbody capacity by 78% paints a similar picture, with significantly stronger reduction compared to Value and ULC carriers.
As a result of the significant capacity cuts, FSCs operated with unit costs averaging 2.9x prior year levels (2.6x for the narrowbody fleet), while Value carriers operated with a peak CASM of 1.5x Q2’19-levels (and 1.6x vs. Q3’19). ULCCs reached a peak unit cost of 1.8x. All this was in spite of fuel prices being down by more than 50% in the latter quarter. The two key drivers for this increase inherently are (1) lower aircraft and crew utilisation and (2) the share of overhead fixed costs prior to the pandemic. FSCs reduced their aircraft utilisation to a mere 2.7 block hours per day in Q2’20, while their competitors were able to keep utilisation at roughly 4 hours/day. The FSCs’ overhead costs made up (pre-pandemic) about 26% of total costs, that of Value carriers and ULCCs stood around 22% and 16% respectively.
From a gauge perspective, North American FSCs leveraged their fleet portfolio to fly with smaller (regional) aircraft (-12% seats/aircraft year on year in Q2’20), while ULCCs lowered it by about 4%, and Value carriers kept it relatively stable.
When it comes to the recovery, in Q2’21, Value carriers and ULCCs appear to be closest to their Q2’19-unit costs. FSCs reached 60% of pre-pandemic capacity levels with a CASM 19% above Q2’19 despite 10% lower fuel cost, while Value carriers operated with 81% of Q2’19 capacity and their CASM remained 4% above Q2’19, despite 12% lower fuel cost. ULCCs reached the same levels of capacity compared to Q2’19 but remained 9% above Q2’19, despite 12% lower fuel cost.
It appears clear that ULCCs and Value carriers were able to manoeuvre through the crisis more cost-efficiently than their FSC peers. While Value carriers and especially ULCCs are almost back to full capacity in Q2’21, FSCs still lag in capacity and cost recovery, partially due to the slower return of intercontinental traffic. A lower share of overhead cost acted as a shield of resiliency for the Value and ULC carriers during the crisis.
Example 2: United’s route competitiveness from NYC
Another dashboard mock-up is presented in chart 4. This dashboard provides detail of route competitiveness from a market and cost perspective. The example displays the route competitiveness of United Airlines’ (UA) routes from NYC, by city-pair, in 2019. The x-axis represents the symmetric logarithmic relative market share (RMS) of UA, ie the market share versus its strongest competitor per route. For example, on the city-pair NYC-SFO, UA had the highest market share, about 2x the share of its strongest competitor.
The y-axis displays the relative cost competitiveness (RCC), ie the unit cost (CASM) of UA against the cheapest competitor on that route. On the route NYC-SFO, the cheapest competitor exhibits about 25% lower unit costs. Since all these values are calculated on a per-route basis, additional information is available to further investigate each route. Hovering over each data point in the dashboard (cannot be visualised here) displays detailed information on seats operated by carrier and aircraft in the selected timeframe as well as the associated unit costs for the carrier-aircraft combination. This supporting data unveils that the second largest operator on this route was Delta, with about 420,000 seats (UA had about 980,000 seats), explaining the high RMS score. On the RCC side, Alaska operated most of its seats (c320,000) with a 737-900 at 8.5¢/ASM. United’s unit costs were between 10-12¢/ASM (depending on the aircraft type), explaining United’s low score on the RCC axis.
Such data is available for every airline and network to help management understand the market positioning with a few simple clicks. Obviously, all airlines aim to have most routes in the top-right quadrant (market and cost leaders) and fewest in the bottom-left quadrant (market and cost lagging routes). Even airlines with relatively high unit costs can identify their sweet spot on each route depending on the aircraft deployed by their competitors and the related unit costs.
Further information
The images in this article cannot reflect the full functionality of skailark’s dynamic dashboards for obvious reasons. For a demonstration of example functionality, two publicly available dashboards providing indexes for unit costs and CO2 data have been created, which can be accessed at skailark.com/airline-cost-index/. The founders of skailark welcome any feedback from subscribers to Aviation Strategy. Live demonstrations of the dashboards can be arranged.